P/NP问题:修订间差异
小 robot Adding: fr, ko |
无编辑摘要 |
||
第4行: | 第4行: | ||
[[计算复杂度理论]]是[[计算理论]]处理解决给定问题所需的资源的一部分。最常见的资源就是时间(解决问题的步数)和空间(需要多少内存来解决问题)。 |
[[计算复杂度理论]]是[[计算理论]]处理解决给定问题所需的资源的一部分。最常见的资源就是时间(解决问题的步数)和空间(需要多少内存来解决问题)。 |
||
在这个理论中,类'''[[P (复杂度)|P]]'''有所有那些可以在一个确定序列化机器上一和输入大小的多项式表达的时间内解决的[[决定问题]]组成;类'''[[NP (复杂度)|NP]]'''有所有其肯定解可以在给定正确信息的多项式时间内验证的决定问题组成,或者等效的说,那些解可以在[[非确定图灵机]]上在[[多项式时间]]内找出的问题的集合。很可能,[[计算理论]]最大的未解决问题就是关于这两类的关系的: |
|||
In this theory, the class '''[[P (complexity)|P]]''' consists of all those [[decision problem|decision problems]] that can be solved on a deterministic sequential machine in an amount of time that is polynomial in the size of the input; the class '''[[NP (complexity)|NP]]''' consists of all those decision problems whose positive solutions can be verified in polynomial time given the right information, or equivalently, whose solution can be found in [[polynomial time]] on a [[Non-deterministic Turing machine | non-deterministic]] machine. Arguably, the biggest open question in [[theory of computation|theoretical computer science]] concerns the relationship between those two classes: |
|||
: |
:'''P'''和'''NP'''相等吗? |
||
在2002年对于100研究者的调查,61人相信答案是否定的,9个相信答案是肯定的,22个不确定,而8个相信该问题可能和现在所接受的公理独立,所以不可能证明或证否。[http://www.cs.umd.edu/~gasarch/papers/poll.ps] 对于正确的解答,有一个[[Clay Mathematics Institute|$1,000,000美元的奖励]]。 |
|||
An important role in this discussion is played by the set of [[NP-complete]] problems (or '''NPC''') which can be loosely described as those problems in '''NP''' that are the least likely to be in '''P'''. (See [[NP-complete]] for the exact definition.) Theoretical [[computer scientist]]s currently believe that the relationship among the classes '''P''', '''NP''', and '''NPC''' is as shown in the picture, with the '''P''' and '''NPC''' classes disjoint. |
An important role in this discussion is played by the set of [[NP-complete]] problems (or '''NPC''') which can be loosely described as those problems in '''NP''' that are the least likely to be in '''P'''. (See [[NP-complete]] for the exact definition.) Theoretical [[computer scientist]]s currently believe that the relationship among the classes '''P''', '''NP''', and '''NPC''' is as shown in the picture, with the '''P''' and '''NPC''' classes disjoint. |
2005年10月18日 (二) 04:25的版本
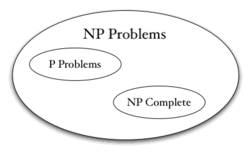
模板参数错误!(代码36)
|
计算复杂度理论是计算理论处理解决给定问题所需的资源的一部分。最常见的资源就是时间(解决问题的步数)和空间(需要多少内存来解决问题)。
在这个理论中,类P有所有那些可以在一个确定序列化机器上一和输入大小的多项式表达的时间内解决的决定问题组成;类NP有所有其肯定解可以在给定正确信息的多项式时间内验证的决定问题组成,或者等效的说,那些解可以在非确定图灵机上在多项式时间内找出的问题的集合。很可能,计算理论最大的未解决问题就是关于这两类的关系的:
- P和NP相等吗?
在2002年对于100研究者的调查,61人相信答案是否定的,9个相信答案是肯定的,22个不确定,而8个相信该问题可能和现在所接受的公理独立,所以不可能证明或证否。[1] 对于正确的解答,有一个$1,000,000美元的奖励。
An important role in this discussion is played by the set of NP-complete problems (or NPC) which can be loosely described as those problems in NP that are the least likely to be in P. (See NP-complete for the exact definition.) Theoretical computer scientists currently believe that the relationship among the classes P, NP, and NPC is as shown in the picture, with the P and NPC classes disjoint.
In essence, the P = NP question asks: if positive solutions to a YES/NO problem can be verified quickly, can the answers also be computed quickly? Here is an example to get a feeling for the question. Given a large number Y, we might ask whether Y is a composite number. For example, we might ask whether the number 53308290611 has nontrivial factors. The answer is YES, though it would take a fair amount of work to find a factor by hand. On the other hand, if someone claims that the answer is "YES, because 224737 is a divisor of 53308290611", then we can quickly check that with a single division. Verifying that a number is a divisor is much easier than finding the divisor in the first place. The information needed to verify a positive answer is also called a certificate. So we conclude that given the right certificates, positive answers to our problem can be verified quickly (i.e. in polynomial time) and that's why this problem is in NP. Although this particular problem was recently shown to be in P as well (see references for "PRIMES in P" below), this is not at all obvious, and there are many other similar problems that are not believed to be in P.
The restriction to YES/NO problems doesn't really make a difference; even if we allow more complicated answers, the resulting problem (whether FP = FNP) is equivalent.
Formal definitions
More formally, a decision problem is a problem that takes as input some string and requires as output either YES or NO. If there is an algorithm (say a Turing machine, or a LISP or Pascal program with unbounded memory) which is able to produce the correct answer for any input string of length n in at most nk steps, where k is some constant independent of the input string, then we say that the problem can be solved in polynomial time and we place it in the class P. Intuitively, we think of the problems in P as those that can be solved reasonably quickly.
Now suppose there is an algorithm A(w,C) which takes two arguments, a string w which is an input string to our decision problem, and a string C which is a "proposed certificate", and such that A produces a YES/NO answer in at most nk steps (where n is the length of w and k doesn't depend on w). Suppose furthermore that
- w is a YES instance of the decision problem if and only if there exists C such that A(w,C) returns YES.
Then we say that the problem can be solved in non-deterministic polynomial time and we place it in the class NP. We think of the algorithm A as a verifier of proposed certificates which runs reasonably fast. (Note that the abbreviation NP stands for "Non-deterministic Polynomial" and not for "Non-Polynomial".)
NP-complete
To attack the P = NP question, the concept of NP-completeness is very useful. Informally, the NP-complete problems are the "toughest" problems in NP in the sense that they are the ones most likely not to be in P. This is because any problem in NP can be transformed, in polynomial time, into an instance of any specific NP-complete problem. For instance, the decision problem version of the traveling salesman problem is NP-complete. So any instance of any problem in NP can be transformed mechanically into an instance of the traveling salesman problem, in polynomial time. So, if the traveling salesman problem turned out to be in P, then P = NP! The traveling salesman problem is one of many such NP-complete problems. If any NP-complete problem is in P, then it would follow that P = NP. Unfortunately, many important problems have been shown to be NP-complete and not a single fast algorithm for any of them is known.
Still harder problems
Although it is unknown whether P=NP, problems outside of P are known. The problem of finding the best move in Chess or Go (on an n by n board) is EXPTIME-complete. Because it can be shown that P ≠ EXPTIME, these problems are outside P, and so require more than polynomial time. The problem of deciding the truth of a statement in Presburger arithmetic is even harder. Fischer and Rabin proved in 1974 that every algorithm which decides the truth of Presburger statements has a runtime of at least 2^(2^(cn)) for some constant c. Here, n is the length of the Presburger statement. Hence, the problem is known to need more than exponential run time. Even more difficult are the undecidable problems, such as the halting problem. They cannot be solved in general given any amount of time.
Is P really tractable?
All of the above discussion has assumed that P means "easy" and "not in P" means "hard". While this is a common and reasonably accurate assumption in complexity theory, it is not always true in practice, for several reasons:
- It ignores constant factors. A problem that takes time 101000n is P (it is linear time), but is completely intractable in practice. A problem that takes time 10-100002n is not P (it is exponential time), but is very tractable for values of n up into the thousands.
- It ignores the size of the exponents. A problem with time n1000 is P, yet intractable. Problems have been proven to exist in P that require arbitrarily large exponents (see time hierarchy theorem). A problem with time 2n/1000 is not P, yet is tractable for n up into the thousands.
- It only considers worst-case times. There might be a problem that arises in the real world such that most of the time, it can be solved in time n, but on very rare occasions you'll see an instance of the problem that takes time 2n. This problem might have an average time that is polynomial, but the worst case is exponential, so the problem wouldn't be in P.
- It only considers deterministic solutions. There might be a problem that you can solve quickly if you accept a tiny error probability, but a guaranteed correct answer is much harder to get. The problem would not belong to P even though in practice it can be solved fast. This is in fact a common approach to attack problems in NP not known to be in P (see RP, BPP).
- New computing models such as quantum computers, may be able to quickly solve some problems not known to be in P; however, none of the problems they are known to be able to solve are NP-hard. However, it should be noted that the definition of P and NP are in terms of classical computing models like Turing machines. Therefore, even if a quantum computer algorithm were discovered to efficiently solve an NP-hard problem, we would only have a way of physically solving difficult problems quickly, not a proof that the mathematical classes P and NP are equal.
Why do computer scientists think P ≠ NP?
Most computer scientists believe that P≠NP. A key reason for this belief is that after decades of studying these problems, nobody has been able to find a polynomial-time algorithm for an NP-hard problem. Moreover, these algorithms were sought long before the concept of NP-completeness was even known (Karp's 21 NP-complete problems, among the first found, were all well-known existing problems). Furthermore, the result P = NP would imply many other startling results that are currently believed to be false, such as NP = co-NP and P = PH.
It is also intuitively argued that the idea of problems that are hard to solve, but easy to verify the solutions for matches our own real-world experience.
On the other hand, some researchers believe that we are overconfident in P ≠ NP and should explore proofs of P = NP as well. For example, in 2002 these statements were made: [2]
- The main argument in favor of P≠NP is the total lack of fundamental progress in the area of exhaustive search. This is, in my opinion, a very weak argument. The space of algorithms is very large and we are only at the beginning of its exploration. [ . . . ] The resolution of Fermat's Last Theorem also shows that very simply [sic] questions may be settled only by very deep theories.
- — Moshe Vardi, Rice University
- Being attached to a speculation is not a good guide to research planning. One should always try both directions of every problem. Prejudice has caused famous mathematicians to fail to solve famous problems whose solution was opposite to their expectations, even though they had developed all the methods required.
- — Anil Nerode, Cornell University
Results about difficulty of proof
Although a million dollar prize and a huge amount of dedicated research with no substantial results is enough to show the problem is difficult, there have also been some formal results demonstrating why the problem might be difficult to solve.
One of the most frequently-cited is a result involving oracles. Imagine you have a magical machine that can solve only one problem, such as determining if a given number is prime, but can solve it instantly. Our new question is now, if we're allowed to use this machine as much as we want, are there problems we can verify in polynomial time that we can't solve in polynomial time? It turns out that, depending on the problem that the machine solves, it can be shown both that P = NP and P ≠ NP. The practical consequence of this is that any proof which can be modified to account for the existence of this magic machine cannot solve the problem. Unfortunately, most known methods and nearly all classical methods can be modified in such a way (we say they are relativizing).
As if this weren't bad enough, a 1993 result by Razborov and Rudich showed that, given a certain credible assumption, proofs that are "natural" in a certain sense cannot solve the P = NP problem.[3] This demonstrated that some of the most seemingly-promising methods of the time were also unlikely to succeed. As more theorems of this kind are proved, a potential proof of the theorem has more and more traps to avoid.
This is actually another reason why NP-complete problems are useful: if a polynomial-time algorithm, or the lack of one, can be demonstrated for an NP-complete problem, this would solve the P = NP problem in a way which is not believed to be excluded by the above results.
Polynomial-time algorithms
No one knows whether polynomial-time algorithms exist for NP-complete languages. But if such algorithms do exist, we already know some of them! For example, the following algorithm correctly accepts an NP-complete language, but no one knows how long it takes in general. This is a polynomial-time algorithm if and only if P = NP.
// Algorithm that accepts the NP-complete language SUBSET-SUM. // // This is a polynomial-time algorithm if and only if P=NP. // // "Polynomial-time" means it returns "YES" in polynomial time when // the answer should be "YES", and runs forever when it's "NO". // // Input: S = a finite set of integers // Output: "YES" if any subset of S adds up to 0. // Otherwise, it runs forever with no output. // Note: "Program number P" is the program you get by // writing the integer P in binary, then // considering that string of bits to be a // program. Every possible program can be // generated this way, though most do nothing // because of syntax errors.
FOR N = 1...infinity FOR P = 1...N Run program number P for N steps with input S IF the program outputs a list of distinct integers AND the integers are all in S AND the integers sum to 0
THEN OUTPUT "YES" and HALT
If P = NP, then this is a polynomial-time algorithm accepting an NP-Complete language. "Accepting" means it gives "YES" answers in polynomial time, but is allowed to run forever when the answer is "NO".
Perhaps we want to "solve" the SUBSET-SUM problem, rather than just "accept" the SUBSET-SUM language. That means we want it to always halt and return a "YES" or "NO" answer. Does any algorithm exist that can provably do this in polynomial time? No one knows. But if such algorithms do exist, then we already know some of them! Just replace the IF statement in the above algorithm with this:
IF the program outputs a complete math proof AND each step of the proof is legal AND the conclusion is that S does (or does not) have a subset summing to 0 THEN OUTPUT "YES" (or "NO" if that was proved) and HALT
Logical characterizations
The P=NP problem can be restated in terms of the expressibility of certain classes of logical statements. All languages in P can be expressed in first order logic with the addition of a least fixed point operator (effectively, this allows the definition of recursive functions). Similarly, NP is the set of languages expressible in existential second order logic — that is, second order logic restricted to exclude universal quantification over relations, functions, and subsets. The languages in the polynomial hierarchy, PH, correspond to all of second order logic. Thus, the question "is P a proper subset of NP" can be reformulated as "is existential second-order logic able to describe languages that first-order logic with least fixed point cannot?"
Trivia
The Princeton University computer science building has the question "P=NP?" encoded in binary in its brickwork on the top floor of the west side. If it is proven that P=NP, the bricks can easily be changed to encode "P=NP!". [4]
Hubert Chen, PhD, of Cornell University offers this tongue-in-cheek proof that P does not equal NP: "Proof by contradiction. Assume P = NP. Let y be a proof that P = NP. The proof y can be verified in polynomial time by a competent computer scientist, the existence of which we assert. However, since P = NP, the proof y can be generated in polynomial time by such computer scientists. Since this generation has not yet occurred (despite attempts by such computer scientists to produce a proof), we have a contradiction."[5]
See also
External links and references
- The Clay Math Institute Millennium Prize Problems
- Gerhard J. Woeginger. The P-versus-NP page. Lists a number of incorrect solutions to the problem.
- A. S. Fraenkel and D. Lichtenstein, Computing a perfect strategy for n*n chess requires time exponential in n, Proc. 8th Int. Coll. Automata, Languages, and Programming, Springer LNCS 115 (1981) 278-293 and J. Comb. Th. A 31 (1981) 199-214.
- E. Berlekamp and D. Wolfe, Mathematical Go: Chilling Gets the Last Point, A. K. Peters, 1994. D. Wolfe, Go endgames are hard, MSRI Combinatorial Game Theory Research Worksh., 2000.
- Neil Immerman. Languages Which Capture Complexity Classes. 15th ACM STOC Symposium, pp.347-354. 1983.
- Computational Complexity of Games and Puzzles
- Manindra Agarwal, Nitin Saxena, Neeraj Kayal, "PRIMES is in P", Preprint, August 6, 2002
- The "PRIMES is in P" FAQ
- Scott Aaronson's Complexity Zoo